We have been using generative AI in our daily lives now.
Be it writing emails, asking queries or generating ideas, we are using them to make the process faster and be more productive.
But do you ever wonder how they work? What are the types of generative AI models?
Let’s understand how these models work and how they are making our lives easy.
To quickly summarize, we will learn:
- What are generative artificial intelligence models?
- How do these models work?
- Primary goal of generative ai model
- Types of Gen AI models
- What type of data is generative AI most suitable for?
What Are Generative AI Models?
Generative AI models are basically a subset of Artificial intelligence. They are designed to respond like humans based on the input that they get. They are a bit different from traditional AI. Traditional AI is trained for recognizing patterns and making decisions. But generative AI focuses on producing new outputs. It includes images, text and audio.
Importance and Applications of AI-Generative Models
Generative AI models have diverse applications across sectors, including healthcare, gaming, design, and media. They’re pivotal in scenarios requiring high-quality synthetic data, creative applications like artwork or music composition, and personalized digital experiences. From generating lifelike images to assisting in natural language processing tasks, their adaptability is driving innovation across multiple domains.
How Do Generative AI Models Work?
Generative AI models mainly operate by recognizing patterns in the inputs that we give them. They run it through the neural network to analyze it. Then they provide outputs based on the input. It makes them very important for tasks like generating data, content and more.
Neural Networks and Deep Learning
Generative AI leverages neural networks. To be more precise, it uses deep learning. It uses layered structures to find complex patterns in data. These deep neural networks helps generative models to capture intricate data features. It makes them very effective for generating realistic images, text and sounds.
Training Methods: Unsupervised and Semi-Supervised Learning
Generative models often rely on unsupervised learning. The models usually explore data without the set outputs. In semi-supervised learning, some portion of output data is still given for analysis. This approach helps AI generate outputs on their own, and this is mainly the reason why we call it generative AI.
Data Sources and Pattern Recognition
Generative artificial intelligence models need a variety of data for learning. It helps them recognize patterns, identify features like object shape in images and sentence structure in text. This improves the overall output and makes them sound more human.
Types of AI Generative Models
1. Variational Autoencoders (VAEs)
VAEs are generative models that use encoder-decoder architectures to compress input data into a latent space and then reconstruct it. By sampling from this latent space, VAEs can generate new, unique outputs. They are particularly useful for applications requiring smooth data transformations and realistic, yet novel, representations.
Explanation of VAEs and Their Architecture
VAEs have two components. First is the encoder and second is decoder. Encoder compresses the input data into lower dimensional latent space and decoder reconstructs this data. The latent space here makes sure that each output is distinct but still resembles the input data.
Training Process and Latent Space Representation
During training, VAEs optimize reconstruction accuracy while ensuring a smooth latent space. This latent space representation makes VAEs ideal for applications in image synthesis, text generation, and anomaly detection.
Use Cases and Examples of VAEs
VAEs are used in fields like image synthesis. It is because they can create realistic images and find irregular patterns in data.
2. Generative Adversarial Networks (GANs)
GANs consist of two neural networks: a generator and a discriminator. Both of them compete against each other. The generator creates new data and the discriminator authenticates it. It helps models learn on their own and produce realistic images.
GANs and Components
There are two main components in GANs. First is the generator, it produces realistic data. And the other one discriminators, it tries to differentiate between the real and generated data. They work against each other and help you get an output that is more realistic.
Training Process and Adversarial Learning
GANs undergo adversarial training, where the generator and discriminator improve through competition, producing increasingly realistic outputs.
Real-World Applications and Breakthroughs with GANs
GANs power applications like deepfake technology. It helps create realistics 3D modeling and high quality images in gaming and media. It is changing how gaming companies create graphics and deliver more immersive experiences.
3. Auto-Regressive Models
Auto-regressive models generate sequences one step at a time, predicting each data point based on prior ones. These models are widely used in natural language processing, as they excel at generating coherent text by learning from vast language datasets.
Auto-Regressive Models and Structure
These models predict each token or pixel by referencing previous ones, making them effective for sequence data.
Training and Inference Process
Auto-regressive models are trained to predict the next element in a sequence. It makes them more effective for generating text.
Use Cases of Auto-Regressive Models
Some of the use cases include language generation, like chatbots and image generation.
4. Flow-Based Models
Flow-based models are generative AI systems that use invertible transformations, making them capable of mapping complex data distributions. They offer exact probability densities, providing greater control over the generation process.
Explanation of Flow-Based Models
These models apply transformations to data that can be inverted, allowing precise control over data generation.
Normalizing Flows and Invertible Transformations
By using normalizing flows, flow-based models offer smooth transformations. It is ideal for applications requiring precise density estimation.
Applications and Advantages of Flow-Based Models
Flow-based models are used in tasks where exact data probability is crucial, such as density estimation and video generation.
5. Transformer-Based Models
Transformer-based models mostly use attention mechanisms for processing long range dependencies in data. It makes them more effective for tasks that involve language, images and audio. They are also used in NLP as they can help with translation.
Explanation of Transformer-Based Models
These models use self-attention to capture dependencies within data, producing coherent and context-aware outputs.
Applications and Advantages
Transformers power language models like GPT and BERT, excelling in text-based applications like chatbots and virtual assistants.
Also read this article : Artificial Intelligence Companies in India
Benefits of Generative AI Models
- Data Augmentation: Generative AI can generate output on its own. This output is unique and it is added to the dataset. So, it helps models learn things on its own with their own outputs.
- Synthetic Data Generation: When real-world data is limited or sensitive, generative models can create similar data to help fill those gaps.
- Natural Language Processing: Generative AI models are very good at generating realistic outputs. It includes images, text and videos. That is the reason why they are used in chatbots, translation and generating content.
- Image and Video Synthesis: Generative models can generate high-quality images or videos. It is really helpful in creative fields like media and design as it quickly generates the media.
- Creative and Artistic Uses: Generative AI models are very helpful for artists. They can help with creating new styles and also provide inspiration for art and more.
- Versatility in Various Domains: One of the best things about generative AI is how flexible it is. Its flexibility makes it a perfect choice for multiple industries.
Challenges in Generative AI Models
- Mode Collapse in GANs: Sometimes these models produce similar outputs over and over again. It limits their creativity.
- Training Complexity: Generative models need a lot of computing power. It makes the training process long and expensive.
- Adversarial Attacks: These models can sometimes be tricked and misused for purposes it is not designed for.
- Fine-Tuning and Transfer Learning: Adapting Gen AI models for different tasks is tricky and complex. It's because you need a lot of data for it. So, it requires extra tuning to work at its best.
Examples of Generative AI Models
- GPT-X (GPT-3, GPT-3.5, GPT-4): We all use it and these models are only getting better with GPT 5 about to be launched in the coming months. <br>
- OpenAI Codex: Again this is designed by OpenAI. It is used for coding and Gitbut Copilot uses this model.<br>
- Stable Diffusion: This is used for generating images for text. It is really helpful for creating digital art. <br>
- LaMDA: It is a transformer based model. It is designed for conversations as it is able to sound more natural and can easily understand the context.<br>
- PaLM: PaLM is more focused on multilingual content generation. It improves AI capabilities and helps reach more audiences worldwide. <br>
- AlphaCode: It is developed by DeepMind, and it helps with code generation and problem solving. <br>
- LLaMA: The main focus of LLaMA is to make large language models easily accessible. It has a smaller and efficient design. So you can use it even with less computing power. <br>
- Midjourney: Midjourney helps generate realistic images using the input prompts. It is known for its artistic designs. <br>
Conclusion
So, these were the generative AI models that are used by most businesses today. If you are planning to use this in your business, connect with our team at RejoiceHub. We are experts in using AI and ML models to build custom solutions for your business. With our expertise in using different models, we can help you improve productivity and save cost.

Written by Utsav Davda( AI/ML Engineer)
Rejoicehub LLP, a top-rated IT service provider, places great value on helping other IT professionals across the board. We are consistently delivering comprehensive and high-quality content and products that provide customers with a strategic advantage to improve, expand, and take their business to new heights by using technology. You might as well find us on LinkedIn, Instagram, Facebook or Twitter.
Related Blogs
Best AI Agents for Business to Improve Productivity in 2025

Pranav Rangwala
AIML & Python Expert
Apr 30, 202514 minWhat is AI in the Automotive Industry: A Complete Guide

Keshav Sharma
AI/ML & Python Expert
Apr 29, 202515 minAI in Gaming Industry: Use Cases, Benefits, and More
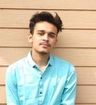
Harsh Mishtry
AI/ML & Python Expert
Apr 23, 202512 minWhat Are the Real-World Uses of AI Agents in Business

Pranav Rangwala
AIML & Python Expert
Apr 18, 202514 minWhat are AI voice agents? A Complete Guide

Keshav Sharma
AI/ML & Python Expert
Apr 17, 202516 minWhat is AI in Marketing? A Complete Guide

Pranav Rangwala
AIML & Python Expert
Apr 9, 202513 minHow Can AI Agents Help Automate Your Workflows in 2025?
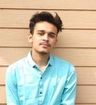
Harsh Mishtry
AI/ML & Python Expert
Apr 7, 202516 minWhat is Agentic AI? Features, Benefits, and More

Keshav Sharma
AI/ML & Python Expert
Apr 2, 202512 minWhat Are Agentic AI Workflows? Benefits, Use Case, and More

Pranav Rangwala
AIML & Python Expert
Mar 31, 202514 minRole and Benefits of Artificial Intelligence in Education
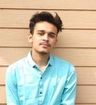
Harsh Mishtry
AI/ML & Python Expert
Mar 26, 202512 minWeekly Newsletter
Get blog articles and offers via email
FAQs
Frequently Asked Questions
Here's a list of FAQs that will help you to know more about our services.
What services does Rejoicehub LLP provide?
How can Rejoicehub LLP help my business with AI/ML?
What is the typical process for a web or mobile development project with Rejoicehub LLP?
How does Rejoicehub LLP ensure the quality of UI/UX design?
What makes Rejoicehub LLP DevOps services different from others?