What is generative AI? Explained Types, Benefits and More

Pranav Rangwala
AIML & Python ExpertGenerative AI has become a hot topic. And with the advent of tools like ChatGPT and Gemini, people are seeing these as harbingers of the age of artificial intelligence. On more practical grounds, the world has only recently discovered their widespread applications and use cases, even though generative AIs date back to the 1960s when Joseph Weizenbaum created the ELIZA chatbot in 1961.
Ever since its inception, generative AI has had many waves of breakthroughs, triggering periods of interest. So, it becomes paramount to get an idea as to what exactly led to a surge, and what possible implications could it bring with it.
What is generative AI?
Generative AIs are deep-learning models that can generate content based on the data they are trained on. This could be text, speech, sounds, images, or any other media. The recent advancements in terms of simplicity and speed have vastly increased their applications. You could ask the AI to write poetry, create a song in the style of your favourite artist, or in any way mimic art to the point that it looks authentic.
The last set of breakthroughs led to advances in computer vision five years ago, and the current advancements in natural language processing have followed, greatly increasing the models' ability to use and understand language. What's even more interesting is that this use extends to things like software code or any other data type.
How does generative AI work?
Generative AI uses machine learning models to learn patterns and relationships in the content it is trained with. IT has the ability to generate new content based on those patterns.
Generative AI models comprise neural networks that recognise patterns and structures in a given set of data to generate content. These are trained with a large amount of unlabeled data, i.e. data that isn't labelled, to identify its characteristics and create what's called foundation models. Foundation models are the base for AI systems, such as GPT-3, that can perform multiple tasks.
1. Training
The training of generative AI begins with training a base model, for example, large language models for text, or equivalent models for image, video, or audio generation. Generative AI models are trained using deep learning on a lot of unstructured data, such as text or media available on the internet. During training, the generative AI model predicts the next element in sequences, for example, the next word in text. Thus, it fine-tunes itself to reduce errors. The result is a neural network that can generate content autonomously based on a prompt. Training requires great computational resources and time.
2. Fine-Tuning
Generative AI foundation models are generalists that need fine-tuning to be specific to a certain task. This will involve adding labeled datasets related to the application, like customer support questions with correct responses for a chatbot. Fine-tuning will increase the accuracy and relevance of the model, although it is often tedious as most of the data used for labeling must be outsourced. Reinforcement Learning with Human Feedback (RLHF) RLHF enhances the performance of generative AI models through human feedback. Users rate or grade outputs, allowing the generative AI model to learn from the corrections and enhance its accuracy or relevance. This is especially useful for fine-tuning interactive generative AI applications, such as chatbots.
3. Generation, Evaluation, and Ongoing Tuning
Generative AI models undergo constant assessment and refinement to enhance their effectiveness. Developers may perform updates every week, making adjustments to enhance accuracy or relevance. Methods such as Retrieval Augmented Generation (RAG) can enrich the generative AI model with external, current information, thereby ensuring improved outputs and greater transparency for users.
What type of data is generative AI most suitable for?
While the variety of data generative AI has proven its proficiency, the different forms of data differ in terms of how suited they are for a generative model. The most suited data types among these are text, data data, image data, time series data, multimodal data, and structural data.
Time-series data can be analyzed to study patterns in historical data for accurate predictions and can be used to predict data such as market trends or maintain contextual relevance. On the other hand, structured data completes and generates synthetic data to augment existing data sets, as in generating new entries or predicting missing values in spreadsheets.
Which technology helped generative AI create convincingly authentic media?
Primarily, 2 technologies led the way in aiding generative AI with the ability to craft media that is convincingly authentic. These consist of: Generative Adversarial Networks or (GANs) and Generative Pre-trained Transformers or (GPT).
Generative Adversarial Networks or (GANs) function using 2 simultaneously trained neural networks. These consist of the generator and the discriminator, and they create new data while evaluating its authenticity.
It is the competition between the two networks that leads to increasingly realistic content. GPT models are based upon the transformer architecture. It uses self-attention mechanisms to process data. With that, GPT models are used for generating text, language translations, text summarisation and answering questions.
Discover how generative AI can transform your business—explore our AI/ML Development Services.
When was generative AI open source?
Open-source generative AI burst onto the scene in 2021. This was when tech companies began sharing their groundbreaking models with the public. One notable example is GPT-2, a language model trained on millions of web pages. This has ignited a passionate debate amongst various leaders of the industry.
But again, through accessibility to all, we can foster innovation while driving progress. That is the main aim of generative AI as a technology. Some of these benefits include:
- Transparency to ensure ethical use
- Collaboration and Innovation on a global scale.
- Accessibility and Cost-Effectiveness are needed in order to make it freely available.
- Safeguarding educational use
- Security through continuous scrutiny
What generative AI can create?
1. Text
Generative AI models, especially those based on transformers, can generate coherent and contextually relevant text. They generate content like blogs, articles, reports, emails, and creative writing while taking care of the mundane work of producing drafts for summaries and meta descriptions, leaving ample time for higher-value tasks.
2. Images and Video
Generative AI tools like DALL-E and Stable Diffusion can create realistic images and original art, and do the job of style transfer and image editing and enhancement. Recently developed AI video tools can create an animation directly from a written text prompt and apply special effects to the video far faster and far cheaper than traditional methods can.
3. Sound, Speech, and Music
Generative AI creates natural-sounding speech for products, such as AI chatbots and virtual assistants, as well as audiobook narration.
But it can also create original music compositions, mimicking the form and style of professional products
4. Software Code
Generative AI creates code, auto-completion, translation between programming languages, and summarizing functionality as some of the ways to support developers. It streamlines prototyping, debugging, and refactoring while providing the user with a natural interface to coding tasks.
5. Design and Art
Generative AI creates unique art and designs or supports graphic design tasks. Applications range from generating characters, avatars, environments, and special effects for virtual simulations video games, and other creative industries.
6. Simulations and Synthetic Data
Generative AI generates synthetic data to be applied in areas, such as drug discovery. It designs molecular structures that have certain properties, creating synthetic environments and datasets to help in research and development.
Benefits of generative AI
1. Improved Creativity
Generative AI inspires creativity as it creates many new, unique variations of content, which can be a good starting point or reference for the writer, artist, and designer. It also overcomes creative blocks and facilitates streamlined brainstorming processes.
2. Better (and Faster) Decision Making
Generative AI analyses huge datasets, unearths patterns, and generates insights to aid in smarter, data-driven decisions. It can produce hypotheses and actionable recommendations for professionals such as executives, analysts, and researchers.
3. Dynamic Personalization
Generative AI tailors content in real-time by analyzing user preferences and history. Applications include recommendation systems and personalized content creation, enhancing user engagement and satisfaction.
4. Constant Availability
Generative AI operates 24/7 without fatigue, enabling uninterrupted support through customer service chatbots, automated responses, and other continuous operations.
What's next for generative AI?
Generative AI is set to undergo future advancements with growing adoption and aggressive ongoing research. The most important change that the worldwide user base would like to see is in the accuracy of the results to ensure the reliability of the tool. We can also expect future versions to have better interfaces and streamlined workflows with customisations in the way we interact with the AI. There is also a lot of scope for improvements in tool integrations.
For programmers, code consistency is a matter of importance, enforcing best practices in coding and formatting. In terms of application: Data Processing: AI in data transformation, labeling, augmented analytics.
- Semantic Web: Mapping internal taxonomies to external job skills.
- Risk Assessments: Transforming third-party data for risk analysis.
- 3D Modeling and Design: AI in product design, 3D modelling.
- Drug Development: Assisting in new drug discovery.
- Digital Twins and Supply Chains: Enhancing business processes, supply chain management.
- Business Innovation: Generating new product ideas exploring business models.
Conclusion
Generative AI, whilst being in its nascent stage, is already transforming industries, and this impact is only set to grow as it improves and its use cases increase. Through constant innovation and aggressive research, generative AI can undergo a series of improvements to facilitate better customizations, enhanced tool integration, and accuracy. Through active collaborations happening worldwide, developers could unlock their future potential while ensuring ethical use. For more information on AI and ML development services at RejoiceHub.
It is exciting to see what the future holds for Generative AI. It is a game-changer and has the potential to forever change how we interact with machines and access the internet. Generative AI is already seeing widespread adoption due to its ease of access and usability. It promises us the likelihood of being the future of work.

Written by Pranav Rangwala(AIML & Python Expert)
Rejoicehub LLP, a top-rated IT service provider, places great value on helping other IT professionals across the board. We are consistently delivering comprehensive and high-quality content and products that provide customers with a strategic advantage to improve, expand, and take their business to new heights by using technology. You might as well find us on LinkedIn, Instagram, Facebook or Twitter.
Related Blogs
What is Simple Reflex Agents in AI?

Keshav Sharma
AI/ML & Python Expert
May 5, 202516 minWhat is AI Automation? All You Need to Know
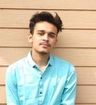
Harsh Mishtry
AI/ML & Python Expert
May 1, 202518 minBest AI Agents for Business to Improve Productivity in 2025

Pranav Rangwala
AIML & Python Expert
Apr 30, 202514 minWhat is AI in the Automotive Industry: A Complete Guide

Keshav Sharma
AI/ML & Python Expert
Apr 29, 202515 minAI in Gaming Industry: Use Cases, Benefits, and More
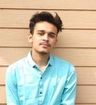
Harsh Mishtry
AI/ML & Python Expert
Apr 23, 202512 minWhat Are the Real-World Uses of AI Agents in Business

Pranav Rangwala
AIML & Python Expert
Apr 18, 202514 minWhat are AI voice agents? A Complete Guide

Keshav Sharma
AI/ML & Python Expert
Apr 17, 202516 minWhat is AI in Marketing? A Complete Guide

Pranav Rangwala
AIML & Python Expert
Apr 9, 202513 minHow Can AI Agents Help Automate Your Workflows in 2025?
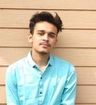
Harsh Mishtry
AI/ML & Python Expert
Apr 7, 202516 minWhat is Agentic AI? Features, Benefits, and More

Keshav Sharma
AI/ML & Python Expert
Apr 2, 202512 minWeekly Newsletter
Get blog articles and offers via email
FAQs
Frequently Asked Questions
Here's a list of FAQs that will help you to know more about our services.
What services does Rejoicehub LLP provide?
How can Rejoicehub LLP help my business with AI/ML?
What is the typical process for a web or mobile development project with Rejoicehub LLP?
How does Rejoicehub LLP ensure the quality of UI/UX design?
What makes Rejoicehub LLP DevOps services different from others?